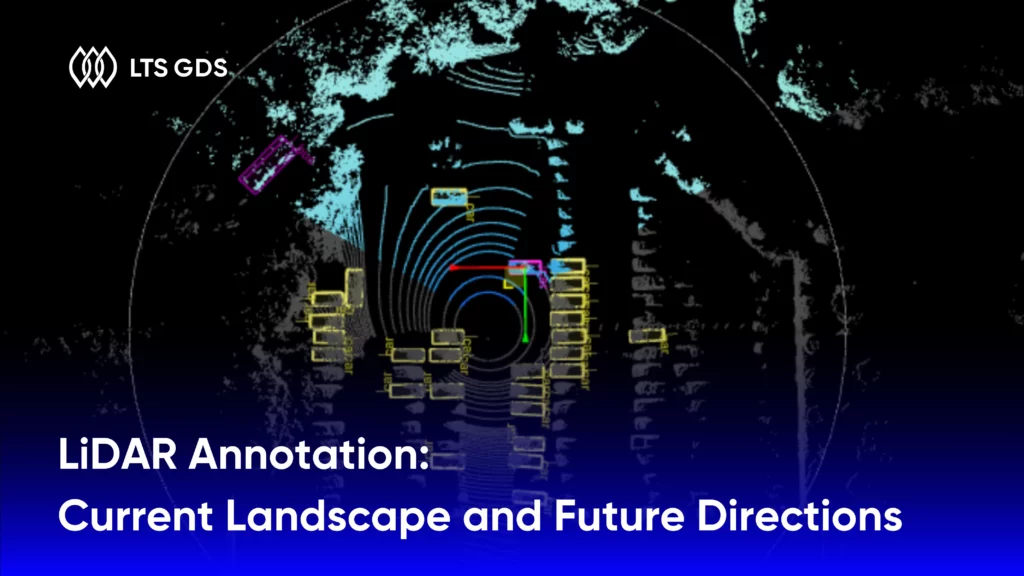
In recent years, the impact of LiDAR technology has been profound, shaping the global technology landscape and significantly benefiting human life. According to Mordor Intelligence, the LiDAR market is projected to reach $2.57 trillion in 2024 and surge to approximately $6.3 trillion by 2029, indicating a remarkable compound annual growth rate (CAGR) of 19.93% during the 2024-2029 period. This rapid growth is fueled by the soaring demand for autonomous vehicles and the widespread application of LiDAR across diverse industries.
So, what exactly is LiDAR technology? How do we annotate data collected by LiDAR sensors? What are the practical applications of LiDAR annotation? Future trends and challenges of LiDAR annotation? In the article below, the information and knowledge about LiDAR annotation will be systematized to provide readers with the most general overview of this data annotation technique.
What is LiDAR Technology?
LiDAR, short for Light Detection and Ranging, is a remote sensing technology that uses light in the form of a laser to measure the distance to an object or surface. This technology works by emitting a laser beam from a source and capturing the light reflected from the object’s surface. The time it takes the laser light to travel from the source to the object and back is used to calculate distance, creating a detailed 3D map of the scanned area.
What is LiDAR Annotation?
LiDAR annotation is the process of classifying and labeling objects in 3D data points collected from a LiDAR sensor. This process is essential for training Machine Learning models, as the quality of the annotated images directly influences the algorithm’s performance. High-quality annotations are crucial for accurate object recognition and environmental understanding.
Essential Types of LiDAR Annotations
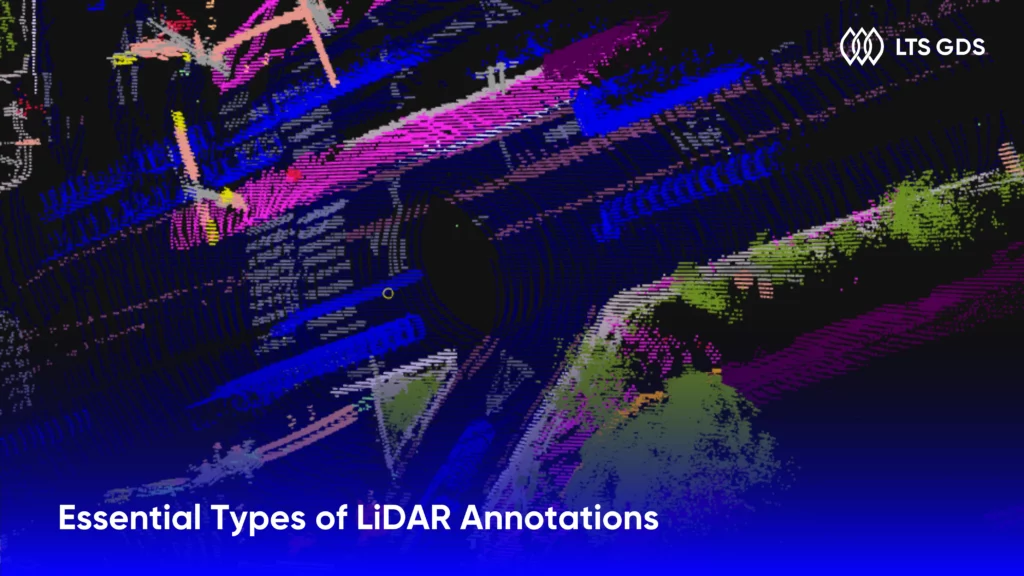
The definition above shows that LiDAR is a complex technology, and annotating the data collected from LiDAR will require accurate annotation methods. Now, let’s explore the common types of LiDAR annotations and their role in different projects.
3D Cuboid
The 3D Cuboid is a cube used to surround an object in three-dimensional space. This type of annotation helps in classifying and determining the position, size, and orientation of objects. It is considered the easiest and fastest annotation method for data images collected from LiDAR. However, 3D Cuboid may not be suitable for detailed and complex annotations of objects like trees, electrical lines, and pipelines.
Semantic Segmentation
Semantic segmentation involves labeling each object or group of objects into classes such as roads, sidewalks, or buildings. It helps in identifying and classifying objects while also providing context about the surrounding environment in 3D, thus improving annotation accuracy.
Using semantic segmentation in LiDAR annotation can be complex and requires expertise. If the data collected from LiDAR is noisy or incomplete, it may affect the annotation results using the semantic segmentation method.
Instance Segmentation
The instance segmentation method not only distinguishes each class like semantic segmentation but also differentiates each separate object within the same class. During the LiDAR annotation process, instance segmentation will be responsible for tracking each object, counting the number, and analyzing the behavior of the objects. Similar to semantic segmentation, the instance segmentation method is complex and requires the annotators to have high technical expertise. Furthermore, this method will make it difficult to accurately identify obscured or overlapping objects.
Point-Level Annotation
This approach is utilized to identify the specific features or structures of objects, often used for recognizing faces, emotions, and behaviors of objects. In situations where LiDAR data is gathered from various sources, the point-level method assists in aligning time, converting coordinate systems, positioning, orienting, and comparing data, thus simplifying analysis and ensuring reliable data applications.
However, the point-level method may pose challenges when used for complex objects. Additionally, as LiDAR data regarding the surrounding environment is regularly updated, annotating using the point-level method can be complex, expensive, and unstable.
Trajectory Annotation
Trajectory involves connecting a series of line segments through nodes, and it is an annotation method that utilizes curved lines to annotate features in image data or three-dimensional spatial data. In the context of LiDAR annotation, the trajectory approach is used to outline linear objects in the landscape such as roads, railway tracks, lanes, electrical lines, rivers, and more. For image data that requires frequent updates like LiDAR, the trajectory can be easily modified to accurately represent changes in the real-world environment. This emphasizes the importance of maintaining the accuracy of images, maps, and spatial data. However, it may not be the most suitable annotation method for non-linear objects, complex shapes, or structures with small details.
Real-Life Applications of LiDAR Annotation
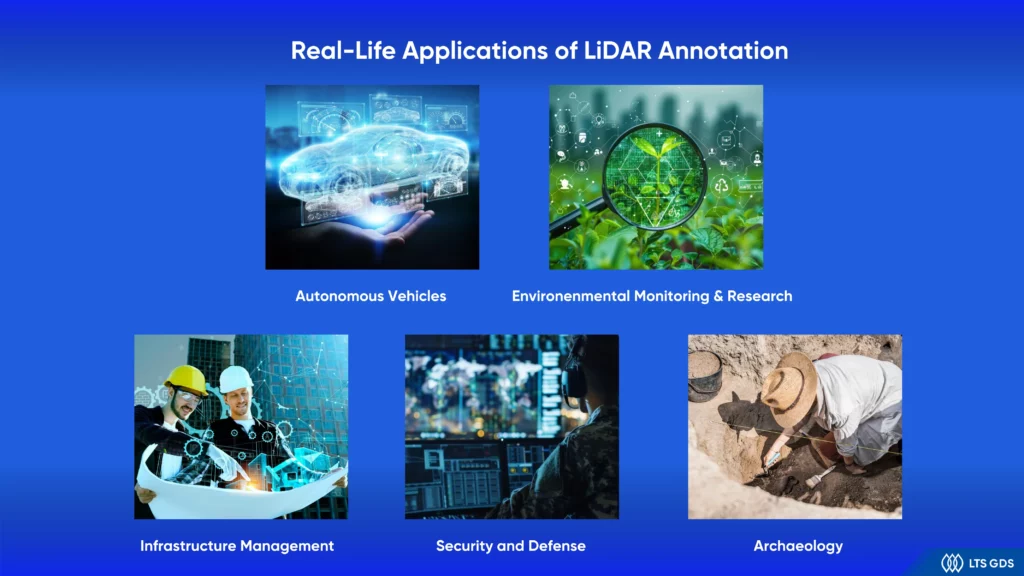
Autonomous Vehicles
Self-driving cars rely on LiDAR, a technology that works by using laser light to scan the surrounding environment and create high-resolution 3D maps. These maps become even more powerful with the addition of multiple annotations, allowing the cars to accurately detect and classify objects such as pedestrians, vehicles, traffic signs, and other road obstacles. With this precise understanding, the vehicles can navigate effectively, make informed decisions, and respond safely to the environment, significantly improving its ability to avoid collisions. In conclusion, LiDAR annotation is the key to unlocking the full potential of autonomous vehicles, ultimately contributing to the realization of a smart transportation future.
Environmental Monitoring and Research
To better understand the role of LiDAR annotation in this field, take forest for example. LiDAR technology utilizes lasers to scan above the treetops, capturing detailed landscape data. Annotating these scans provides researchers with essential information, such as tree heights, species distribution, and growth patterns, which are crucial for assessing forest biodiversity. Moreover, LiDAR annotations further assist researchers in predicting natural disasters by accurately identifying high-risk areas, thereby developing contingency plans, empowering planners to utilize resources efficiently and making informed decisions that promote a healthy balance in natural habitats.
Infrastructure Management
LiDAR annotations have various practical applications, including the management of infrastructure and urban development. By utilizing LiDAR technology, accurate 3D maps of the surrounding area can be created, enabling the development of 3D maps of infrastructure such as roads, bridges, buildings, and utility systems. Annotating this image data can assist urban planning professionals in effectively managing assets, supporting the design and construction of new buildings, and ensuring proper maintenance of infrastructure elements, leading to the successful execution of development projects.
Security and Defense
In the security and defense field, adopting LiDAR technology provides security agencies with critical terrain, route, and military infrastructure data. Annotating these images can assist security agencies in border surveillance, analyzing strategic locations, detecting unusual activities, and swiftly responding to emergencies. Furthermore, LiDAR annotation can enhance the training and readiness of military forces by simulating combat scenarios based on real-world terrain data.
Archaeology
LiDAR annotation is gradually revolutionizing archaeology with its ability to detect, study, and preserve ancient relics. To be more specific, LiDAR technology can scan large areas at high resolution, helping to detect structures buried underground, obscured by terrain, or hidden beneath vegetation. Annotating LiDAR data enables archaeologists to precisely locate and model these relics, facilitating detailed analysis of the structures and its surroundings. Going beyond discovery, LiDAR annotation also affirms its essential value in helping archaeologists predict how relics deteriorate, thereby providing timely conservation measures to protect precious heritage.
Emerging Direction in LiDAR Annotation
High-quality LiDAR Annotation
As the use of LiDAR annotation expands into practical applications, the demand for high-quality LiDAR data annotation is expected to rise, particularly in the field of autonomous driving. This is because precise and thorough annotation of LiDAR data is essential for ensuring the safe and efficient operation of autonomous vehicles, providing competitive advantages to companies operating in the same industry. Therefore, companies can achieve success in their field by focusing on advanced techniques and collaborating with highly specialized engineers to ensure the highest quality of annotated image data.
Read more: How to have high-quality data annotation for Machine Learning?
Automation and AI-Assisted Annotation
In the upcoming years, one of the trends in LiDAR annotation is the increased use of automated and AI-assisted techniques to speed up the process, minimize human intervention, and improve the accuracy of annotated data. Additionally, deep learning models like Convolutional Neural Networks (CNN) and Recurrent Neural Networks (RNN) will be employed to automate tasks such as object detection or semantic segmentation. However, automated annotation will always have the potential for errors, requiring project supervisors to be proactive in quality assurance to ensure the accuracy and detail of the output results.
Semi-supervised and Unsupervised Learning
These techniques will leverage small amounts of labeled data combined with unlabeled or partially labeled data to train Machine Learning models more effectively. In LiDAR annotation, applying these techniques will help detect hidden objects, reducing the effort and cost of manual annotation. Nevertheless, like other automatic annotation techniques, the application of semi-supervised and unsupervised learning requires a human review process to maintain consistent output and prevent unnecessary omissions.
4D Annotation
This is considered an emerging trend in the field of Machine Learning and Artificial Intelligence. This annotation process involves labeling objects in three-dimensional space as well as adding a time dimension to track the trajectory and movement of objects. In practical fields such as autonomous driving, the application of this annotation method will improve the ability to recognize and predict the behavior of moving objects such as pedestrians or vehicles, thereby making safer driving decisions.
The Use of Synthetic Data
Synthetic data is information generated by algorithms, designed to simulate the characteristics and structure of the original data. In a context where real data may be limited or difficult to collect, this trend will contribute to securing a diverse range of LiDAR data inputs and optimizing real-world collection processes, ultimately reducing time expenditures. It can be seen that using synthetic data will also help comprehensively train models, helping to improve understanding and analysis of the surrounding environment. On the other hand, users of synthetic data must diligently verify the information to ensure its accuracy, reliability, and suitability for their intended purposes.
Challenges in LiDAR Annotation
Data Volume
LiDAR data files usually consist of millions to billions of individual data points. Companies that manually annotate LiDAR data must invest a significant amount of time, human resources, and strict management processes to ensure quality and high-efficiency precision.
Complexity of 3D Objects
In a three-dimensional space environment, objects come in various shapes, ranging from simple ones like spheres or boxes to more complex ones like trees, buildings, or vehicles. Some LiDAR data files may contain overlapping or obscured objects, and annotating these complex 3D objects requires significant time and effort as well as efficient processing software and algorithms.
Cost
The cost has a significant impact on the LiDAR annotation process in various ways. Apart from the initial expenses on hardware and equipment, companies relying on software for processing most LiDAR data files need to consider licensing, update, and maintenance costs. On the other hand, companies opting for manual LiDAR annotation should focus on personnel expenses, as this process demands highly skilled annotators and significant human resources to handle large data files.
LiDAR Annotation and the Path to the Future
LiDAR annotation is a challenging process, requiring high precision and accuracy to handle the large volume of image data and the complexity of 3D objects. However, this complexity also reflects the great potential of LiDAR in practical applications. Specifically, in advanced driving assistance systems (ADAS), the LiDAR annotation process not only improves the capability to analyze and process automated systems but also paves the way for future advancements in self-driving vehicle technology.
At LTS Global Digital Services, we are proud to be one of the leading companies providing data annotation services in Vietnam as well as in the Asia-Pacific region, and a trusted partner of customers from markets such as the US, Germany, Korea, and Japan. We are also one of the first companies in the world to achieve ISO/IEC FDIS 5259-4 Certification from DEKRA, confirming the quality of data annotation services that LTS Global Digital Services provides for the AI system and especially for advanced driving assistance systems (ADAS).
Contact us now for further information!