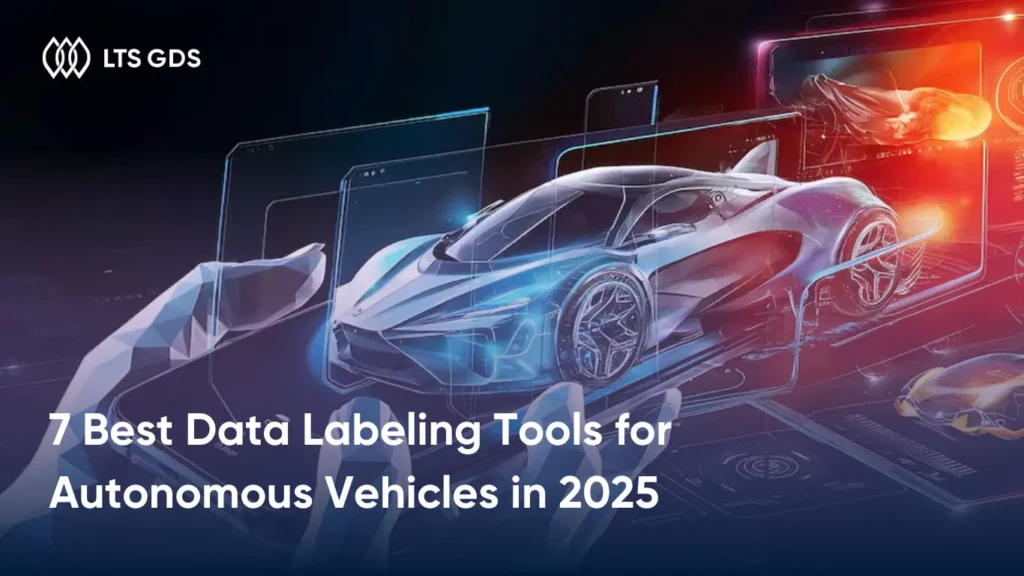
The rise of autonomous vehicles has revolutionized the automotive industry. At the heart of this innovation lies autonomous vehicle data labeling tools, enabling machines to interpret their surroundings. For companies seeking cutting-edge solutions, selecting the right data labeling tool is vital for developing safe and efficient self-driving systems.
In this blog, we’ll explore the role of data labeling tools, their applications in the automotive industry, top tools on the market to enhance your AI-driven projects, and so much more.
What’s a Data Labeling Tool?
A data labeling tool is software that annotates data – images, videos, and sensor readings – to make them usable for machine learning models. In the autonomous driving sector, the quantity and categories of data that need to be labeled are huge and complicated with different types of formats, requiring the use of labeling tools, with specialized features to optimize work efficiency and speed up model development.
Labeled data enables artificial intelligence (AI) algorithms to identify, classify, and predict objects in real-world environments – a critical requirement for autonomous driving systems.
Read more: What is Data Annotation?
The Role of Data Labeling Tools for Autonomous Vehicles
Data labeling tools/platforms play a crucial role in preparing training data with the utmost annotation quality to adapt to the strict requirements of autonomous driving systems.
With semi-automation features that help pre-label and suggest annotations, these platforms streamline the labeling process, enhancing both efficiency and accuracy. These annotations empower autonomous vehicles to perceive and interpret their environment accurately, allowing for informed and reliable decision-making on the road.
Read more: The Role of Data Annotation in the Automotive Industry
Key Applications of Data Labeling Tools in the Automotive Industry
Data labeling software drives critical applications for developing autonomous vehicles, including:
- Self-driving car development: Annotating datasets to train machine learning models for object detection.
- Advanced driver-assistance system (ADAS): Enhancing lane-keeping, adaptive cruise control, and collision avoidance systems.
- Simulation and testing: Generating synthetic data to test vehicle performance in diverse scenarios.
- Traffic analysis: Improving road safety by analyzing traffic flow and patterns.
Each application relies on precisely labeled data to ensure reliable decision-making.
Also read: How to Have High-quality Data Annotation for Machine Learning?
Key Features to Consider When Choosing a Data Labeling Tool
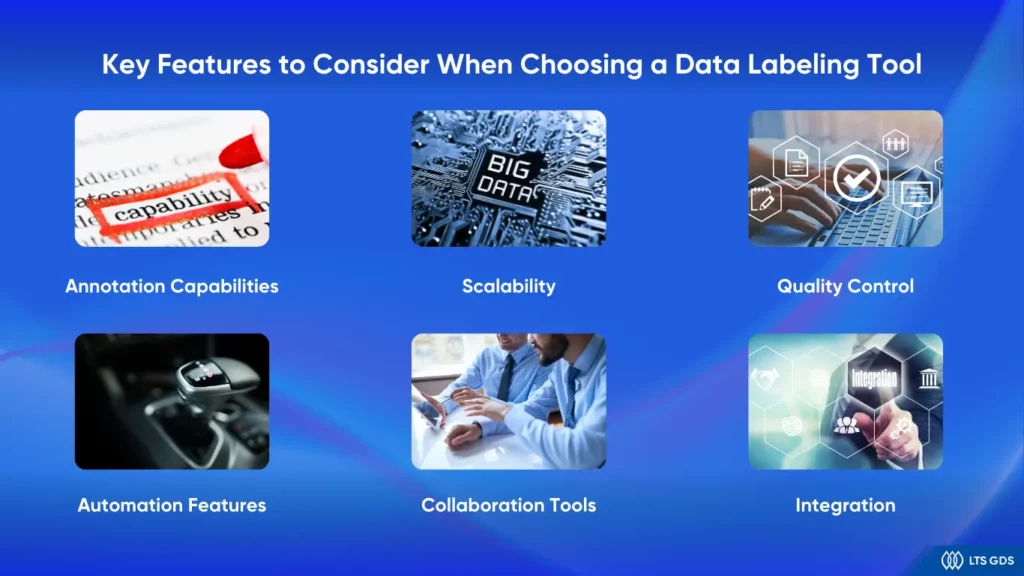
When selecting a data labeling software to process data for developing autonomous vehicles, focus on these key features:
- Annotation capabilities: Support multiple types of labeling like bounding boxes, polygons, keypoints, and 3D annotations.
- Scalability: Ability to manage and process large datasets efficiently.
- Automation features: AI-assisted labeling to reduce manual effort and increase speed.
- Collaboration tools: Features that support seamless teamwork.
- Integration: Compatibility with machine learning workflows and frameworks.
- Quality control: Features to validate and ensure high-quality annotations.
Prioritizing these features will help companies choose the most suitable data labeling tools for their trustworthy AI projects.
Top 7 Data Labeling Tools for Autonomous Vehicles
Here are seven leading data labeling solutions designed for autonomous vehicle development, along with their benefits and limitations:
1. V7
Benefits:
- AI-assisted annotation: Automates annotations to reduce manual effort and save time.
- Advanced features: Supports semantic segmentation, object tracking, and 3D annotation.
- User-friendly interface: Intuitive design improves workflow efficiency.
- Scalability: Manages large datasets effectively for enterprise-level projects.
Limitations:
- Pricing: Advanced features require a paid plan, which may not suit small teams.
- Customization: Limited options for specialized workflows or integrations.
2. Computer Vision Annotation Tool (CVAT)
Benefits:
- Open-source: Free to use, ideal for research teams and small businesses.
- Flexible features: Supports bounding boxes, polygons, polylines, and image classification.
- Scalability: Handles large datasets with robust performance.
- Community-driven: Benefits from contributions by a large developer community.
Limitations:
- Manual labeling: Lacks AI-assisted features for faster annotations.
- Usability: The interface can be unintuitive, requiring training.
- 3D limitations: Not suitable for advanced LiDAR data labeling.
3. SuperAnnotate
Benefits:
- Hybrid annotation: Combines manual and AI-assisted labeling for precision.
- Collaboration tools: Enables team-based workflows with customizable roles.
- Quality control: Built-in review tools ensure high-quality annotations.
- Scalability: Handles projects of all sizes, from startups to enterprises.
Limitations:
- Pricing: Can be costly for smaller teams.
- Learning curve: Advanced features require training for new users.
4. Encord
Benefits:
- Video annotation: Designed for large-scale video projects essential for autonomous vehicles.
- Automated data labeling: AI-powered tools streamline annotation and management.
- Scalability: Handles massive datasets efficiently.
- Project management: Simplifies task organization for teams.
Limitations:
- Training required: Teams need time to learn the data labeling platform.
- Cost: Expensive for small businesses or short-term projects.
5. Labelbox
Benefits:
- Versatile labeling data for machine learning: Supports 2D/3D annotations, video, and sensor fusion.
- AI-assisted tools: Speeds up the labeling process.
- Performance tracking: Analytics to measure and improve workflows.
- Quality assurance: Built-in validation tools for accuracy.
Limitations:
- Pricing: Can be costly for smaller projects.
- Internet dependency: Requires online access, limiting offline use.
6. Roboflow
Benefits:
- End-to-end solution: Offers labeling, augmentation, and model training in one platform.
- Ease of use: Intuitive interface simplifies workflows.
- AI-powered tools: Automates annotations to boost productivity.
- Integration-friendly: Compatible with popular frameworks like TensorFlow and PyTorch.
Limitations:
- Limited 3D support: Not ideal for advanced LiDAR data.
- Customization gaps: May need extra integrations for niche projects.
7. BasicAI
Benefits:
- 2D and 3D annotation: Supports LiDAR, video, and sensor fusion data.
- Specialized tools: Tailored for autonomous driving projects.
- Quality control: Built-in validation ensures consistent accuracy.
- Cost-effective: Competitive pricing for advanced features.
Limitations:
- Community support: Smaller user base compared to open-source data labeling tools.
- Interface design: Usability could be improved for smoother navigation.
Read more:
Top 9 Data Annotation Companies in Asia – Pacific Region
How to Choose the Best Data Annotation Outsourcing Company?
Conclusion
Top data labeling tools for autonomous vehicles are essential for advancing self-driving technology. From object recognition to sensor fusion, these tools help the annotation process take place more effectively and accurately. Selecting the right data labeling tool can accelerate AI development and enhance vehicle performance.
At LTS Global Digital Services, we specialize in delivering excellent data labeling services with extensive experience in utilizing various data tools, including those mentioned above, and managing numerous ADAS-related projects for major clients from South Korea, Europe, and the United States. Our expertise in data annotation and project management ensures precise and efficient solutions for autonomous vehicles. Visit us to learn how we can support your AI initiatives.